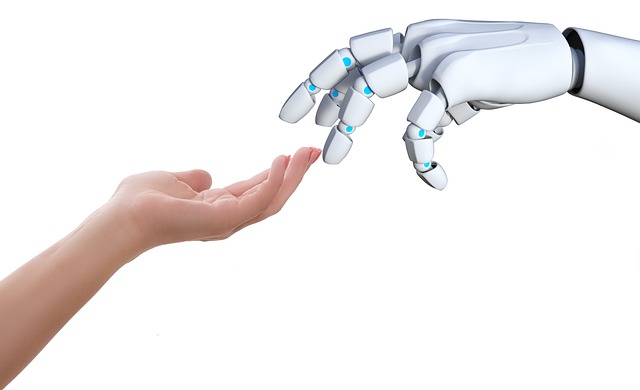
One of the key trends we mentioned in our predictions for 2023 was the rise of the use of augmented analytics.
This is making use of AI to prepare datasets, analyse it, identify trends and patterns and visualise it.
This then leaves the work of the user to interpret and take direct action to influence positive outcomes for the business.
So, this suits not only small companies, who don’t necessarily have the budget to invest in big analytical teams, but equally large companies where the sheer amount of data can just overwhelm.
There are three components to Augmented Analytics:
- Machine learning (ML) which is a type of AI that uses algorithms to analyse datasets identify patterns and deviations
- Natural language technologies, which interprets human language into computer code and vice versa
- Automation of manual routine tasks.
Some of the suggested tools to use are here
Benefits of augmented analytics include:
- Improved decision making: By highlighting trends and equally changes in those trends that can then be investigated by analytical resource to action
- Shift in culture: With more people being able to self- serve around the business it can positively influence culture change to be more data driven.
- Efficiency: By automating the data discovery and manipulation side of things, which a lot of analysts spend a large proportion of their time doing, it means they can focus on the real added value of the ‘So what?’
- Improved predictions: By using AI to understand patterns and create machine learning models, it can predict what will happen next in the trends. This can help with creating budgets and responding to those predicted trends.
This all sounds like the ideal world for any business, so what are the challenges?
- Complexity: Users need a good platform and tool to make full use of the science behind it, without that there will be limited adoption within the business
- Data literacy: There needs to be a good level of understanding of how to understand the output to make the right decision in the business, so there is a need for education.
- Data quality: This is generally the key to analytics but with poor data quality it would limit what the AI and ML algorithms can identify and predict.
Most companies want to move towards being more data driven in their decision making, but often have quite a journey to get to that position due to internal processes, immature data strategy, prioritising the right questions and having the right resource.
As alluded to here, with the challenges of data quality, a fundamental starting point is getting the data in the right position for either human resource or AI and ML to interrogate.
Howard introduced his Hierarchy of Attribution needs to enable action within attribution modelling:
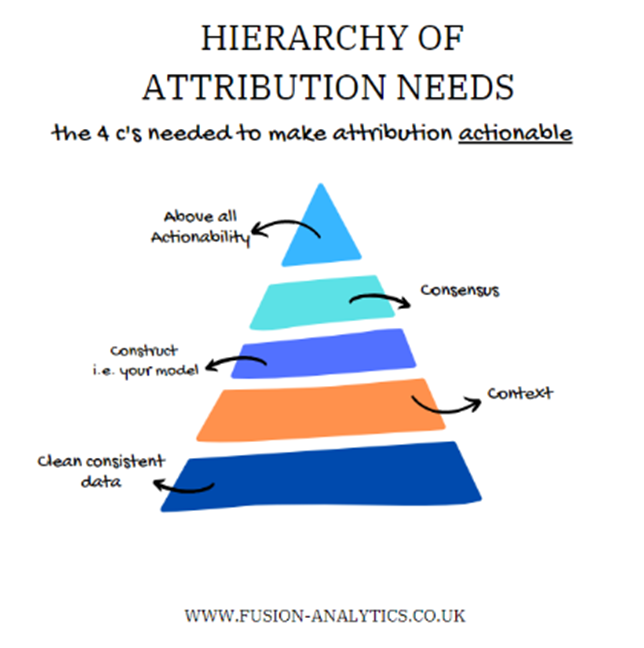
This principle applies whether embarking on an attribution model or developing a suite of dashboards. You need to get the Clean consistent data layer.
If you need help with building that clean consistent data starting point then get in touch.