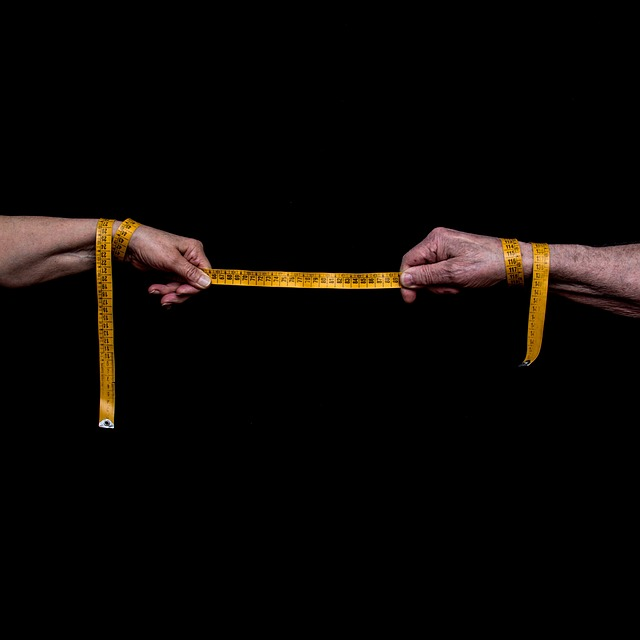
I have sat in many meetings with people (even researchers who normally would run a survey to prove this) who say that “people browse instore and buy online”.
A digital marketing manager for a furniture retailer asked me to look for proof of the reverse. We looked at two approaches; a research based one and online/offline tracking solution which showed that 28-31% of people who bought instore had seen a digital ad within the leading 3months to purchase.
In some cases, we saw that individuals had made over 1000 unique website visits before buying instore!
Similarly, we have worked with a well-known sportswear brand who had product owners, that would argue that products had their own life-cycles and online offline conversations were pointless as people would “find a way” to buy their products.
When we think organisationally about these types of companies, you therefore have to consider:
- Marketing channel & brand owners as functions of spend and demand
- Ecommerce & retail managers as functions of sales and fulfilment
- Product owners; which could be the units of demand and sales
Each of these areas will have independent measurement processes, to prove the ROI & value of their activity, however there will also be high levels of crossover of their insights and metrics.
This means that planning & measurement can quickly go horribly wrong. For example, if we have a new product launch co-inside with new retail store openings, high marketing budget on the same day; all parties can claim the uplift in revenue as related to them. So, we need approaches to help understand how to fairly split the results between them.
For this we refer to our HDDN framework.
The HDDN framework, helps teams align teams around a single plan, for the scope of this article, let’s consider what measurement frameworks we might want to use. For this we need to consider the following areas:
- What methods & KPIS are used to measure each stakeholder’s area of business?
- What are the potential areas for overlap, and do we have a way to quantify these?
- How can we then agree on an aligned plan?
What methods and KPIs are used to measure each stakeholder’s area of business?
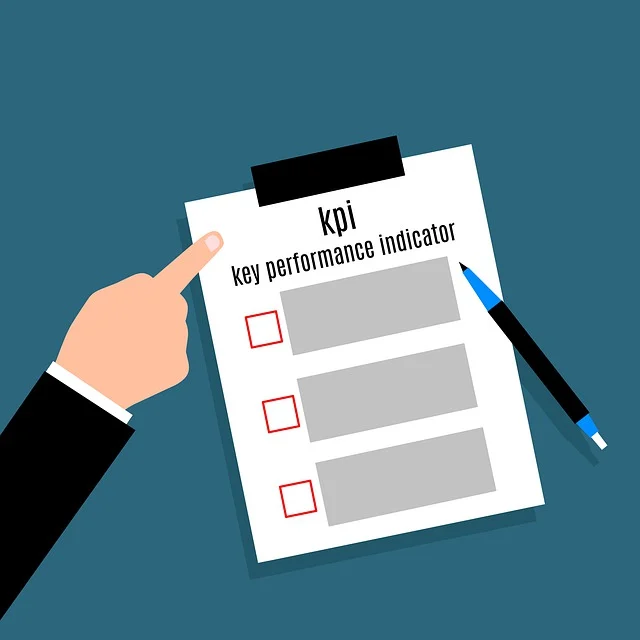
Each team will usually have some claim on revenue or sales as a function of their work.
However, the driving functions are often different, and even how we quantify sales can be different.
A great example was a merchandising team buying products for company, that would feed into their PPC campaigns. Higher value products were often prioritised as they drove a higher initial AOV and therefore ROI on the campaign.
However, CRM teams noticed that the LTV for lower value products was usually higher, as those customers had a higher chance of coming back.
In these cases, whilst the two teams could use different metrics to report, we could align easily align them – enabling optimisation across product and CRM/LTV.
When we compare retail to ecommerce, there will be different versions of similar KPis. For example, sales net of returns can be different if we include the postal cost of free returns for ecommerce.
Whilst there may be totally different methods of measuring marketing activity against some level of value (i.e in store promotions etc), in most cases it can be reasonable to make small adjustments (like how to consider returns) to better align different departments.
It is also important to note that different measurement approaches have different purposes. In the online world most departments use some form of attribution, which focuses on looking at the touchpoints that lead to a sale.
Promotional spend in store can be measured in a similar way (i.e how many units sold) usually it is compared to a non-promotional period (or another store) to confirm the uplift in sales/value; or future purchase rate.
When we start to review other departments models it is important that we accept their findings, whilst trying to understand how and why that might fit in to what we see too.
What are the potential areas for overlap, and do we have a way to quantify these?
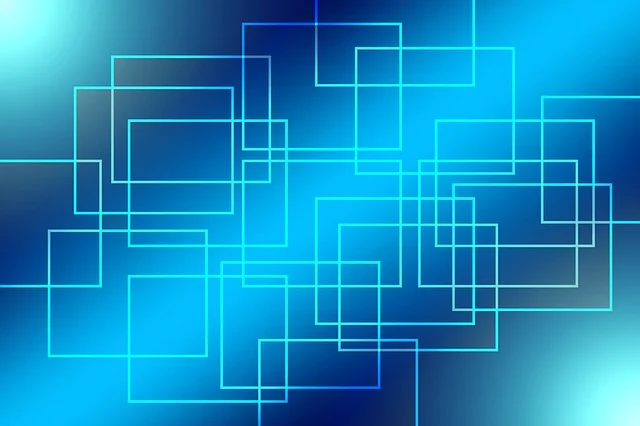
In other cases, it is not so easy to align teams.
For organisations with ecommerce & retail, many performance channels, i.e. digital, are targeted purely on driving online transactions.
Whilst brand is measured on uplift of online + retail sales.
However, we know that this isn’t true given our furniture example of 28% of people seeing and digital ad and buying in store.
In a fully optimised budget, it is understood that channel spend has diminishing returns, i.e. as you increase spend in a channel the ROI tends to reduce (in a channel you get the most benefit up front). Therefore, whilst it makes decisioning easier to have clearly define ROI metrics; it may not be optimal for the business.
It is important to have a view on the impact of online marketing:
- In offline sales
- In the context of new products
- Supported by brand advertising
For these cases we should have a way to measure:
- The overall size of cross over/engagement (i.e. the how many people have bought instore and engaged online)
- The uplift of the marketing activity on its own & when working with others
- Synergies between channels, and which combinations work best together.
Retail sales will have a range of metrics in terms of sales per sq ft etc, however these can also be easily crossed with online marketing.
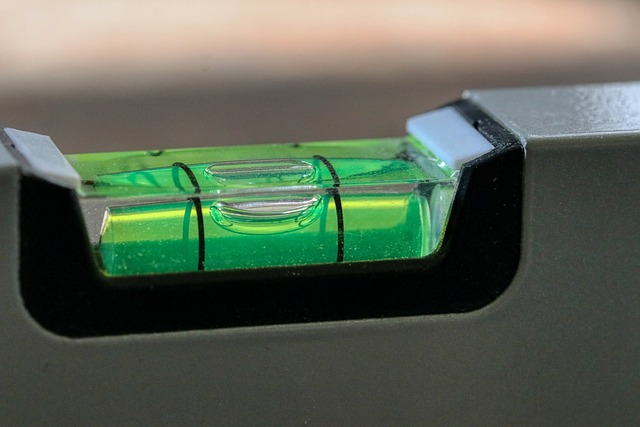
This is where analytics and modelling meets people and process.
As a business we need to align our different departments, however this is tricky when they have their own ways of measuring success.
If we have adopted our first two approaches, to share the measurement approaches and identify areas for overlap, the first step I would take, is to try to ensure that where possible we use the same KPIs.
For example:
- Are we using the same value metrics?
- Do we all consider spend in the same way, how do we consider store promotional spend when comparing to marketing spend?
- Are products categorised in the same way?
- Do we have the same segments or rules to compare groups?
Often, we will see that these items are not aligned. For example, brand spend/return is usually measured in incremental uplift, whilst PPC return is measured in attributed response.
The question here is can we reallocate and brand spend to ppc or ppc sales to brand in order to have a fairer view?
In the case of segments, you may find that there are a number of types of segments used across the business. In retail they may use demographics, whilst online/crm may use RFM metrics (recency frequency value).
Whilst these both have their place in campaign planning; when it comes to overall business value, ideally we would have an agreed single view.
In the areas where there is contest, i.e. does PPC spend drive retail sales or do people browse in store and buy online? then it is often best to refer to standalone pieces of insight to identify the range of potential impacts.
Say for example, we believe that 25-30% of people that buy in store have seen an online AD prior, we may have a corresponding piece of data that says 40-50% of orders online were driven by instore activity.
We can build a plan to:
- On paper understand what the range of impacts mean
- Design tests, or data capture approaches to come up with a more robust assumption
Whilst more work upfront, in getting stakeholders to have conversations and agree on relationships – perhaps doing their own analysis & testing, it does mean that there is a clear framework for decisions, leading to an overall more efficient process.
Impact of AI
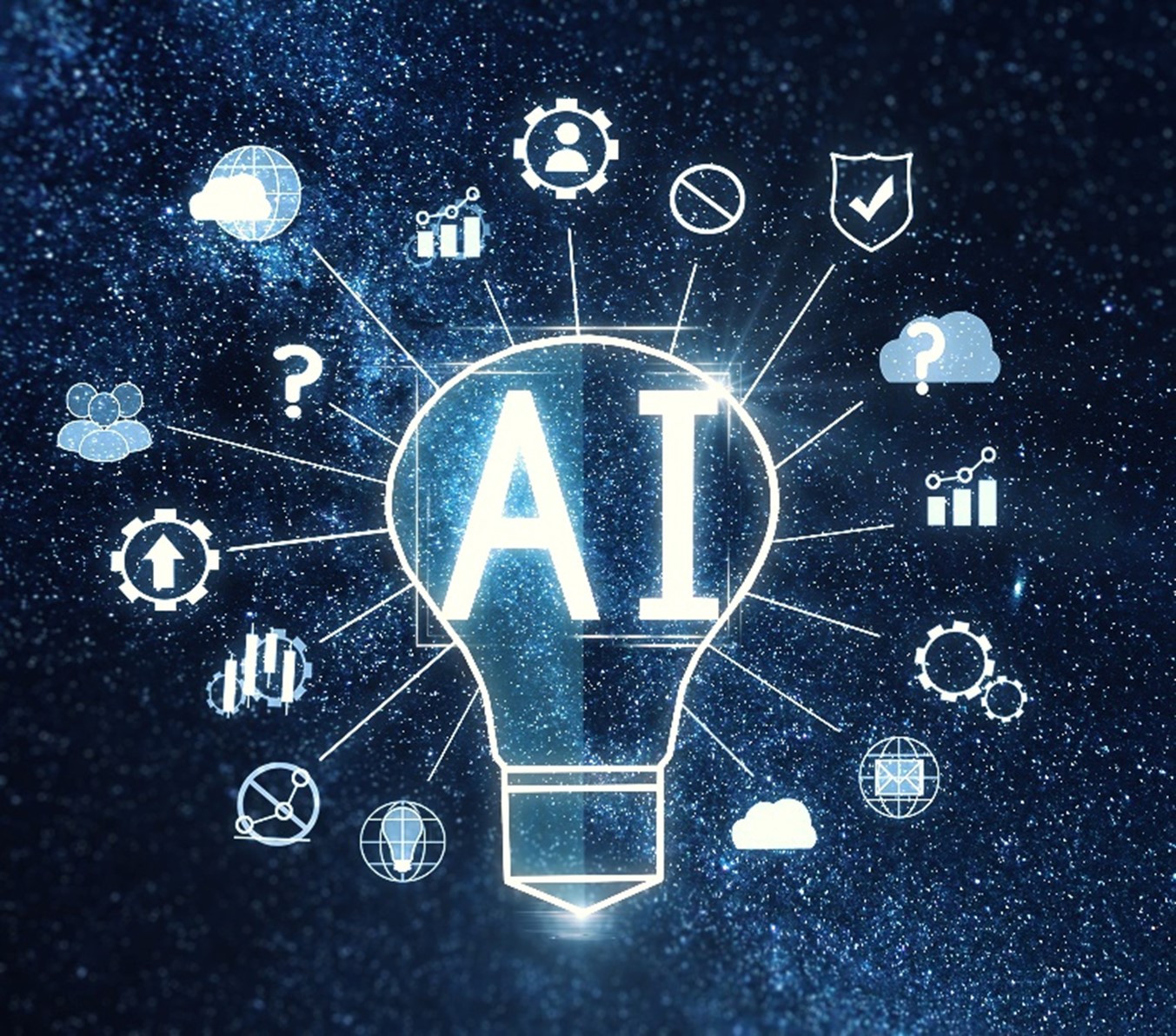
The promise of new insights and models from AI or other ML type platforms, should always be approached with caution.
Anyone can present an answer back with a suggested plan, or numbers back; what you invest in is what is actually the thought process & truth behind those numbers.
Any solution which present findings without detail on “how” it gets to the answer is going to have used a range of assumptions. Whilst it may sound cool to have a model with x hundred or thousand assumptions underneath, as you build your plan on these errors will multiple & you will get further from the truth.
Instead consider using AI to support the identification of good data sources, drivers, or new considerations for planning.